The Essential Role of Data Labeling in Machine Learning
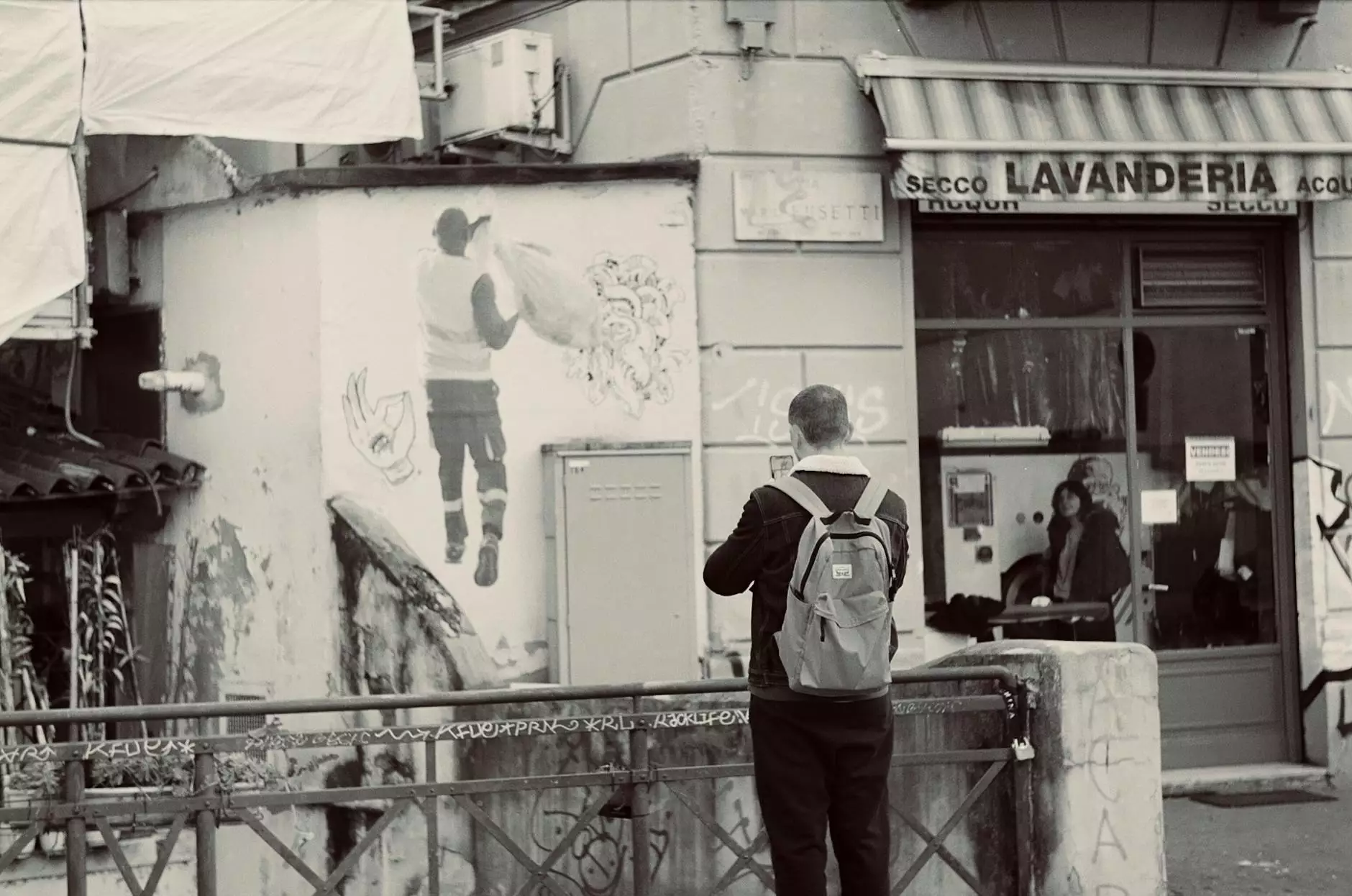
Understanding the intricacies of data labeling machine learning is crucial in today's data-driven landscape. As businesses increasingly lean on artificial intelligence (AI) and machine learning (ML) technologies, the demand for high-quality labeled data continues to surge. This article delves deep into the significance of data labeling, its techniques, and the platforms available to aid organizations like KeyLabs.ai in harnessing the full potential of their data.
What is Data Labeling?
Data labeling is the process of annotating data with informative tags to make it usable for machine learning algorithms. The effectiveness of machine learning models largely hinges on the quality of the data they are trained on. In this context, labeled data refers to datasets that have been annotated with specific tags that define the characteristics of the data. This is a foundational step in building predictive models.
The Importance of Data Labeling
The absence of sufficient labeled data can severely limit the capabilities of a machine learning model. Labeling improves the model's ability to learn and predict outcomes effectively. Here are several key reasons why data labeling is critical:
- Enhanced Model Accuracy: Labeled data allows ML algorithms to learn from examples, leading to higher accuracy in predictions.
- Optimal Training: Machines require well-curated data to train effectively, which helps in improving the overall performance.
- Better Decision-Making: With accurate data, businesses can make informed decisions that drive growth.
- Facilitates Real-World Applications: From self-driving cars to voice assistants, accurate labeling is essential for practical AI implementations.
Types of Data Labeling Techniques
Data can be labeled using several techniques, each suited for different types of data and requirements. The choice of the technique can influence the quality of the final data set. Here are some common methods:
1. Manual Labeling
Manual labeling involves human annotators reviewing data and providing labels. This is often used for complex data types where contextual understanding is necessary. While it can produce high accuracy, it is also time-consuming and costly.
2. Automated Labeling
In this method, algorithms are employed to label data automatically. While faster, this method may struggle with nuanced data where human insight is necessary. It is usually complemented with manual checking for higher accuracy.
3. Semi-Automated Labeling
This technique combines both manual and automated methods, leveraging the strengths of each. Initial labeling can be performed by algorithms, followed by human validation, thus enhancing efficiency and accuracy.
Data Annotation Tools: Essential for Machine Learning Projects
Choosing the right data annotation tool is vital for businesses seeking to label high volumes of data efficiently. KeyLabs.ai offers a variety of tools designed to streamline this process:
Features of KeyLabs.ai Data Annotation Tool
- User-Friendly Interface: Intuitive design that allows for easy navigation and usage by teams of all technical levels.
- Customizable Labels: Users can define specific labels that align with their project requirements, ensuring more accurate annotations.
- Collaboration Features: Multiple users can work on projects simultaneously, enhancing productivity.
- Scalability: Suitable for projects of any size, whether small datasets or extensive databases.
Why Choose a Data Annotation Platform?
A dedicated data annotation platform, such as KeyLabs.ai, provides several advantages over traditional labeling methods. Here are some noteworthy benefits:
1. Efficiency and Speed
Platforms streamline the annotation process, reducing time-to-market for AI solutions. Automated processes significantly cut down on the labor required for manual labeling.
2. Quality Assurance
Many data annotation platforms incorporate rigorous quality checks to ensure the accuracy of the labeled data. This increases the reliability of the training models.
3. Cost-Effectiveness
By outsourcing or automating the labeling process, businesses can cut down significantly on overheads related to hiring large teams for manual labeling.
4. Access to a Pool of Annotators
Data annotation platforms often have networks of skilled annotators, providing access to a broad talent pool for both simple and complex datasets.
The Future of Data Labeling in Machine Learning
The landscape of data labeling is continually evolving, largely driven by advancements in technology and AI. Here are some emerging trends to watch:
1. AI-Assisted Labeling
As machine learning technology advances, AI-assisted labeling is gaining traction. These tools can provide recommendations and learn from user inputs, effectively improving over time.
2. Crowdsourcing
Crowdsourcing annotation tasks to a large pool of users can effectively manage massive datasets, combining the speed of automated tools with the accuracy of human judgment.
3. Evolving Standards and Guidelines
With the growing importance of ethical AI, establishing standards and frameworks for labeling practices will become increasingly crucial. This will help ensure the integrity of data and algorithmic fairness.
Implementing an Effective Data Labeling Strategy
Implementing a successful data labeling strategy involves several key steps:
1. Define Project Goals
Understanding what you want to achieve with your machine learning model will guide your data labeling process and selection of tools.
2. Select the Right Tools
Investing in the right data annotation tool or platform is essential to enhance productivity and data quality.
3. Train Your Team
If you are opting for manual annotation, training your team on the intricacies of accurate labeling is crucial to maintain consistency and quality.
4. Continuously Monitor and Iterate
Monitoring the labeling process, reviewing feedback, and making adjustments as needed will help maintain high-quality labeled data over time.
Conclusion
In conclusion, the world of data labeling machine learning is vital for any organization looking to harness the power of AI. By understanding the importance, utilizing efficient tools and platforms, and staying informed on the latest trends, businesses can enhance their data annotation processes. As the demand for quality labeled data grows, platforms like KeyLabs.ai pave the way for future success in machine learning projects.
Contact Us
Interested in exploring how our data annotation tool and data annotation platform can help your business? Visit KeyLabs.ai today!