Mastering Image Labeling for Object Detection: A Comprehensive Guide
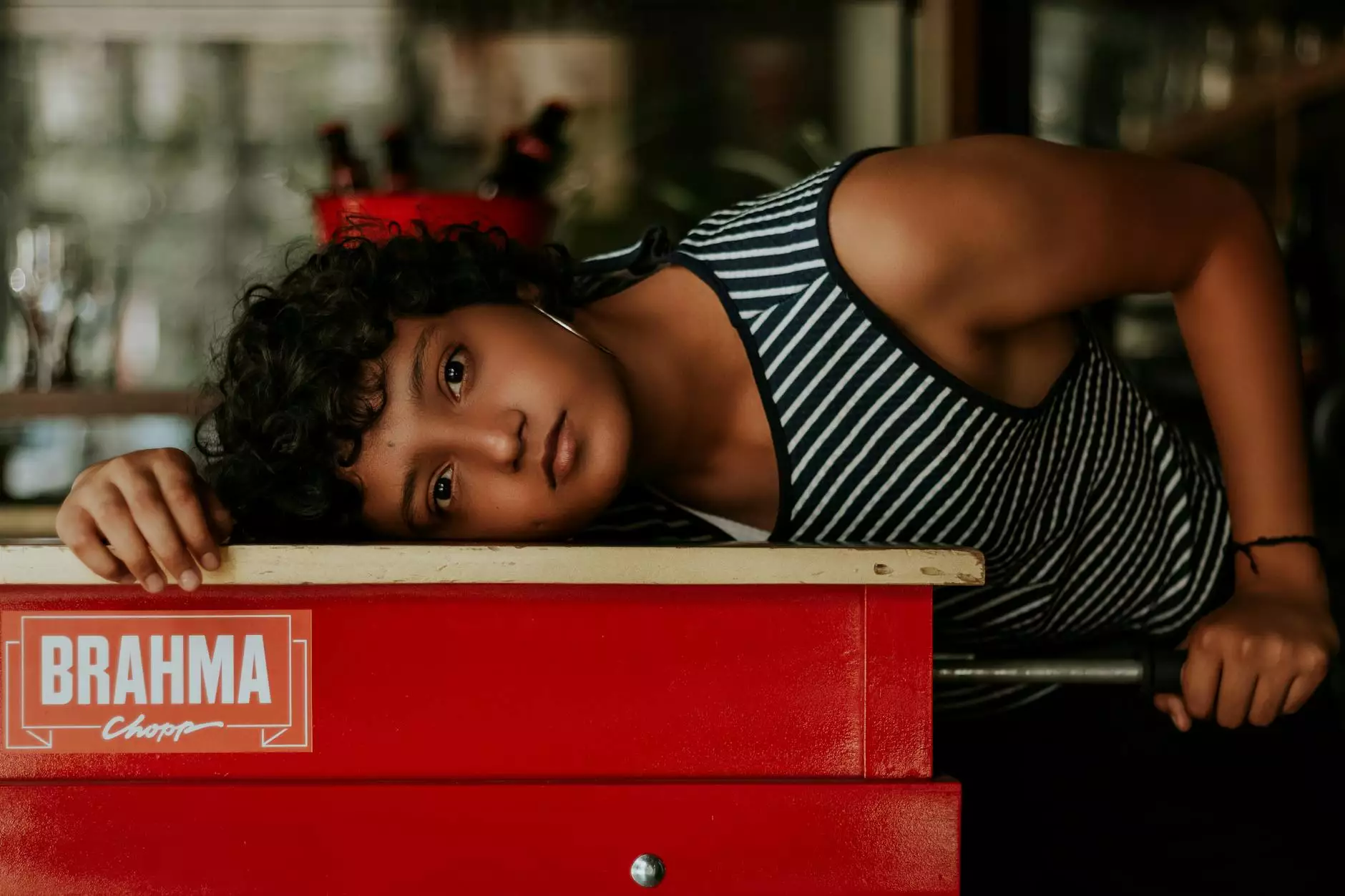
In the age of artificial intelligence and machine learning, the importance of effectively labeling images for object detection cannot be overstated. As businesses increasingly rely on automation for decision making, impeccable data annotation for machine learning becomes critical. This article explores the nuances of data annotation, the tools available, and the best practices to enhance your object detection models, ultimately driving superior outcomes in your business endeavors.
Understanding Object Detection
Object detection is a computer vision task that involves identifying and locating objects within images. It consists of two main components:
- Classification: Determining the category of an object in an image.
- Localization: Identifying the exact position of the object, typically represented by bounding boxes.
The first step to achieving these tasks effectively is proper image annotation. By implementing robust labeling techniques, firms can enhance their machine learning models, leading to improved operational efficiency and better customer experiences.
Why Labeling Images for Object Detection Matters
Labeling images accurately is the backbone of successful machine learning models. Here are some key reasons why it is crucial:
- Data Quality: High-quality labels ensure that the machine learning model can learn effectively, resulting in better predictions.
- Model Accuracy: Accurate labeling directly influences the precision of object detection algorithms, making it vital for applications in fields like autonomous driving, retail, and healthcare.
- Scalability: The faster and more accurately you can label data, the quicker your models can be trained and deployed.
- Cost-Efficiency: Avoid costly misclassifications by ensuring your data is accurately annotated from the start.
Best Practices for Labeling Images for Object Detection
To ensure you are utilizing the best practices in the field of image labeling, consider the following strategies:
1. Use the Right Annotation Tools
The selection of annotation tools is pivotal. Opt for platforms that provide intuitive interfaces, and offer support for various data formats. KeyLabs.ai provides a robust Data Annotation Tool, focusing on efficiency and effectiveness in image labeling. It allows users to:
- Annotate quickly with a variety of tools such as bounding boxes, polygons, and segmentation.
- Collaborate seamlessly with team members to improve labeling throughput.
- Integrate with machine learning models for iterative improvements.
2. Establish Clear Annotation Guidelines
Creating standardized guidelines for annotators is essential. These guidelines should clearly define:
- Labeling conventions (e.g., when to use bounding boxes versus polygons).
- Handling of occluded or overlapping objects.
- Instructions for labeling similar or dissimilar objects.
3. Quality Control and Review Processes
Implement a robust quality assurance framework. This could involve:
- Random checks of labeled images against the original data to ensure accuracy.
- Utilizing feedback loops to continuously enhance the annotation process.
- Encouraging peer reviews among annotators.
4. Invest in Training for Annotators
Providing comprehensive training for all annotators is a game-changer. Proper training helps ensure that your team:
- Understands the importance of high-quality annotations.
- Knows how to use annotation tools effectively.
- Is familiar with domain-specific nuances related to the data.
5. Embrace Automation Where Possible
Utilizing automation tools can significantly expedite the annotation process. If your models already have some predictive capabilities, semi-automated labeling processes can:
- Speed up the initial labeling phase, allowing human annotators to correct and refine the automated labels.
- Reduce the resource burden on teams, letting them focus on more complex annotations.
Choosing the Right Data Annotation Platform
As mentioned earlier, selecting a suitable Data Annotation Platform is fundamental to successful image labeling. Here’s what to look for:
- User-Friendly Interface: Ensure that the platform is intuitive and easy to navigate.
- Integration Capabilities: The platform should seamlessly integrate with your existing ecosystems for a streamlined workflow.
- Scalability: Choose a solution that can grow with your business needs, whether you’re working with hundreds or thousands of image datasets.
- Support and Maintenance: Opt for companies that offer responsive customer service and regular updates to their software.
Conclusion: Unlocking the Potential of Image Labeling
In conclusion, labeling images for object detection is a multifaceted challenge that requires strategic planning, thorough execution, and continuous improvement. The tools and practices outlined in this article provide a roadmap for businesses looking to improve their annotation processes, ultimately leading to more effective machine learning models.
By selecting the right data annotation solution, establishing sound practices, and investing in your team's training, you can set the stage for success in implementing AI-driven initiatives within your organization. Don’t underestimate the power of quality data – it’s the lifeblood of your machine learning projects and can significantly influence your competitive edge in the marketplace.
Visit KeyLabs.ai to learn more about our cutting-edge data annotation tools and how they can help enhance your object detection capabilities today!
label images for object detection